AI and Machine Learning Failures in Banking
Artificial Intelligence and Machine Learning Failures in Banking
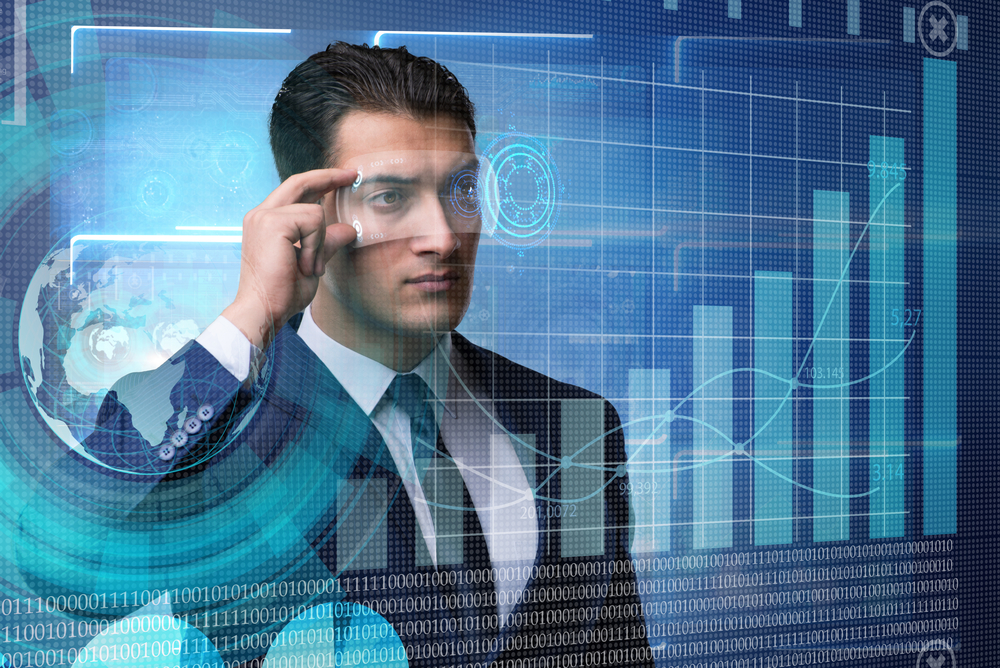
In recent years, Artificial Intelligence (AI) and Machine Learning (ML) have become buzzwords across all industries, including the banking sector. AI and ML are transforming the way banks operate, from customer service to risk management. However, just like any other technology, AI and ML are not immune to failures. In this blog, we will explore some of the bank failures in AI and ML and their potential causes.
One of the most notable bank failures related to AI and ML was the 2012 Knight Capital Group (KCG) debacle. KCG was a market-making firm that used algorithms to trade equities. However, a coding error in one of KCG’s algorithms caused the firm to lose $440 million in just 45 minutes. The algorithm was designed to trade equities, but due to a coding error, it started buying and selling options that KCG did not own. This resulted in significant losses for the firm and eventually led to its bankruptcy.
Another notable bank failure related to AI and ML was the 2019 incident involving Capital One. Capital One is one of the largest banks in the US, and in July 2019, it announced that it had experienced a data breach that affected 100 million customers. The breach was caused by a misconfigured firewall in the bank’s cloud infrastructure. The misconfiguration allowed a hacker to gain access to sensitive customer data, including names, addresses, credit scores, and Social Security numbers. The hacker was able to access this information because Capital One had used an AI-based tool to scan its cloud infrastructure, but the tool failed to detect the misconfiguration.
These are just two examples of bank failures related to AI and ML, but there are several others. So what are some of the potential causes of these failures? One possible cause is a lack of understanding of the technology. AI and ML are complex technologies that require significant expertise to implement and manage. If banks do not have the necessary expertise, they may not be able to properly design, test, and maintain their AI and ML systems, which can lead to failures.
Another potential cause of bank failures related to AI and ML is bias. AI and ML systems are only as good as the data they are trained on. If the data used to train an AI or ML system is biased, then the system will be biased as well. This can lead to decisions that are discriminatory or unfair, which can have significant consequences for banks and their customers.
Finally, inadequate testing and validation can also be a potential cause of bank failures related to AI and ML. Testing and validation are critical to ensure that AI and ML systems are working as intended. However, banks may not be performing enough testing or may not be validating their systems properly, which can lead to unexpected failures.
In conclusion, AI and ML are transforming the banking sector, but they are not immune to failures. Bank failures related to AI and ML can have significant consequences for banks and their customers. To avoid these failures, banks need to ensure that they have the necessary expertise, avoid bias in their systems, and perform adequate testing and validation. By taking these steps, banks can harness the power of AI and ML while minimizing the risks associated with these technologies.